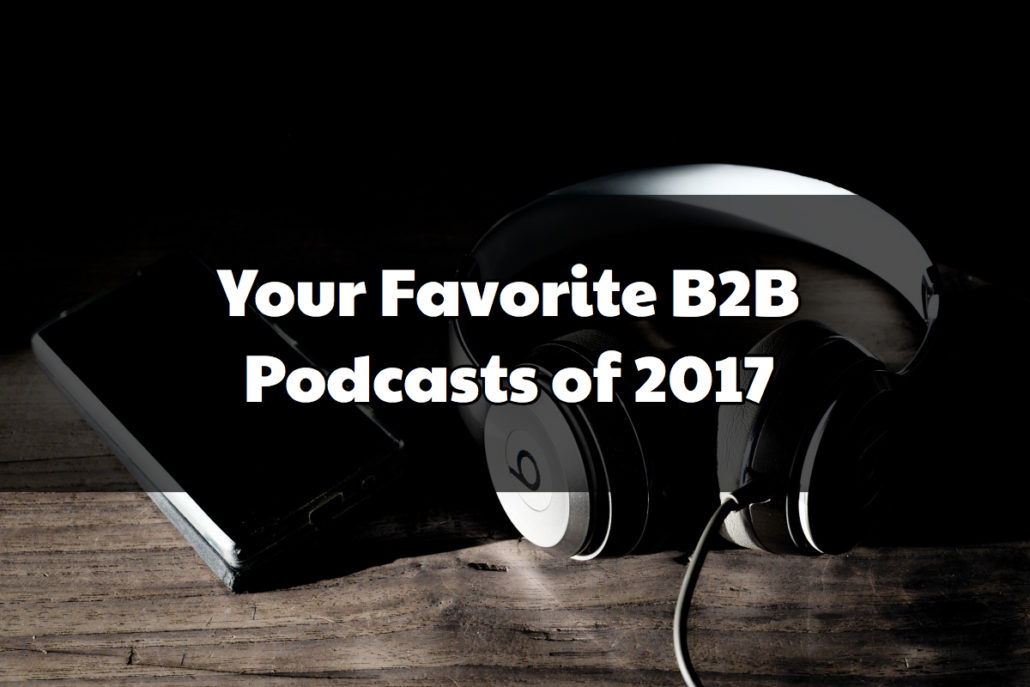
Your Favorite B2B Podcasts of 2017
The B2B Market Research Podcast became B2B Revealed. Our focus broadened to a myriad of issues that impact our clients in the B2B technology sector.
Explore our customer case studies to see how we drive innovation and success in the B2B tech market."