Synthetic respondents or “synths” are artificially created profiles that can simulate the traditional human interaction that takes place in a market research study. These custom profiles are based on comprehensive datasets that can include a combination of public information, bespoke research efforts, and/or other pertinent sources of information.
These synthetic respondents can then go on to serve as participants in market research studies and provide organizations with a deeper understanding of their key research questions. When utilized in conjunction with real respondents, synthetic respondents can contribute valuable insights that have the potential to greatly enhance a company’s marketing, sales, product development efforts, or their overall business strategies.
Benefits of Using Synthetic Respondents in Research
Incorporating synthetic respondents as an augmentation to market research projects offers several benefits, including:
Enhanced Preparation and Refinement
Synthetic respondents offer a range of benefits in the pre-research phase of market research projects. To start, they help researchers with question development and refinement by generating an array of potential questions based on their research objectives. They can also analyze potential questions for clarity, potential bias, and their likelihood of eliciting meaningful responses.
Synthetic respondents can also help to sharpen the study’s focus right from the start, ensuring it zeroes in on the most pertinent and influential topics and target personas. Synthetic respondents, when utilized properly, can stand in as model profiles that represent ideal target audiences, incorporating factors such as demographics, interests, and behaviors.
Finally, synthetic respondents can assist in refining the research methodology. Through preliminary interactions with synthetics, a researcher can experiment with various research designs, sampling techniques, and analytical methods. This experimentation helps to identify the approaches that are most likely to enhance the study’s outcomes, ensuring the research methodology is both effective and efficient.
Cost-effectiveness
Utilizing synthetic respondents in addition to traditional participants presents a cost-effective approach for conducting market research. By replacing a portion of human respondents with synthetic respondents, companies can cut down on the expenses tied to recruiting and compensating non-synths.
While there are initial costs involved in collecting the necessary data to develop synthetic respondents, this early investment can result in significant savings in the long run. By integrating insights from both synthetic and traditional respondents, businesses can achieve a balance, reducing research costs without compromising the quality and reliability of the data collected.
Speed and Efficiency
Leveraging synthetic respondents allows for the rapid refinement of initial research concepts, facilitating the presentation of more refined ideas to human participants. This ensures that the concepts being tested are not only well-developed but also sharply focused. In essence, synthetics give researchers a laboratory of sorts to experiment within, safely, appropriately, and in ways that enhance the entire research effort from start to finish.
This leveraging of a laboratory, full of “synths,” can result in a streamlined research process leading to faster completion of research projects, thereby letting a business gain access to critical business insights that much faster.
Engaging Presentation of Findings
Synthetic respondents can transform the analysis and presentation of research findings into a more dynamic and engaging process, moving beyond the constraints of traditional methods such as slide decks or reports.
For example, synthetic respondents can be generated at the conclusion of a research study, with their profile based on the data and insights collected to date. Doing so allows a set of stakeholders to “chat with the data” in paradigms that are becoming more familiar each day as organizations and individuals embrace technologies such as ChatGPT. Ultimately, by engaging with synths, after a research effort is complete, stakeholders can better understand the nuances of the data and explore various outcomes based on questions that may arise weeks or months after a traditional readout.
Risks Associated with Using Synthetic Respondents in Research
While using synthetic respondents in a market research study offers numerous benefits, it also comes with risks. Researchers need to be aware of:
Avoiding Bias Introduced by Synths
Relying too heavily on synthetic respondents can compromise the integrity of traditional market research, risking biased results. Recent tests revealed that synthetic respondents exhibit biases and lack the diversity and subtlety found in qualitative and quantitative analyses.
Therefore, it is crucial to corroborate synthetic findings with real human feedback and quantitative data. This cross-verification process is essential to enhance the accuracy of research outcomes and reduce bias, ensuring that the insights derived are both reliable and representative of the target population.
Diversity, Equity, and Inclusion
AI models can exhibit biases due to the origins and composition of their training datasets, which are frequently not transparent. When these datasets fail to comprehensively represent a diverse array of demographics, cultural backgrounds, and behaviors, the AI’s outputs can be biased, leading to skewed outcomes.
An illustrative example of this issue is the use of the Common Crawl dataset for training Large Language Models (LLMs). Common Crawl, a vast dataset collected from the internet, is a popular source for training AI due to its size and breadth. However, its composition reveals significant imbalances in language representation; for instance, English content makes up approximately 45% of the dataset, while Polish, among other languages, constitutes less than 2%. This disparity in language representation can result in AI models that are more adept at understanding and generating English content, potentially marginalizing non-English languages and the cultures associated with them.
Without deliberate efforts to include a broad and representative range of data, AI systems risk perpetuating existing biases and creating outcomes that do not fairly or accurately serve the global community.
Privacy and Security Concerns
When synthetic respondents are trained on LLMs, there is a risk of accidental inclusion of private or NDA-protected data into public datasets. If that confidential information is inadvertently incorporated, it can lead to breach of confidentiality, legal and financial repercussions, and concerns about data integrity and security.
This is particularly concerning for businesses and individuals who entrust sensitive data to systems that utilize synthetic respondents. The unauthorized disclosure of private information can damage relationships, tarnish reputations, and lead to a loss of trust in the entity responsible for the data breach.
To address privacy and security concerns, it’s essential for organizations to implement stringent data governance and security measures. This includes conducting thorough data audits, anonymizing personal information, and ensuring that the data used for training synthetic respondents is devoid of sensitive content. Moreover, it’s critical that organizations creating synthetic users maintain ownership or at least control over the models produced. This control ensures that the synthetic respondents can be managed, updated, or corrected in alignment with evolving data privacy standards and organizational needs, thereby safeguarding the integrity and confidentiality of the data involved.
Predictive Limitations
Synthetic respondents, by their nature, cannot experience the present moment as humans do, which may limit their ability to forecast future trends accurately. Unlike real human interviews, which can address emerging issues that might have happened as recently as today, “synths” may not be able to meaningfully comment in these scenarios.
This limitation is partly because synthetic respondents tend to be based on models that rely on historical data, which inherently cannot include the very latest developments. For example, as of March 2024, the publication date of this article, systems similar to ChatGPT would not have access to information or trends that emerged after that time.
Jeff Bezos once emphasized the significance of anecdotal evidence over data when predicting future trends, stating, “When the data and anecdotes disagree, the anecdotes are usually right.” This perspective underscores the value of obtaining human experiences that are based in the present and close observations of these experiences in real time.
Moreover, most LLMs, including those capable of processing text and images, still fall short of the human ability to integrate a wide range of sensory inputs—such as audio, vision, touch, and spatial awareness—into their understanding and their training data sets.
The film “Good Will Hunting” offers a great analogy for this limitation. In one scene, Robin Williams’ character says, “ So if I asked you about art, you’d probably give me the skinny on every art book ever written. Michelangelo, you know a lot about him. Life’s work, political aspirations, him and the pope, sexual orientations, the whole works, right? But I’ll bet you can’t tell me what it smells like in the Sistine Chapel. You’ve never actually stood there and looked up at that beautiful ceiling; seen that.” – Jack Bowen, CEO, Coloop
This analogy highlights a fundamental gap between synthetic respondents and human experiences. While synthetic models can provide comprehensive analyses based on extensive datasets, they lack the depth of perception that comes from direct, multisensory engagement with the world.
Navigating the Risks of Using Synthetic Respondents in Market Research
Employing synthetic respondents in market research offers innovative opportunities for data collection and analysis. However, to effectively navigate the associated risks, researchers must adopt a comprehensive and cautious approach. Below are key strategies to mitigate these risks:
Combine Research Methods
Combining both synthetic respondents and traditional research methodologies is crucial for a balanced and comprehensive analysis. This hybrid approach allows researchers to leverage the efficiency and scalability of synthetic respondents while grounding their findings in the rich, nuanced insights that traditional research methods provide. By doing so, researchers can achieve a more accurate and holistic understanding of their subject matter, ensuring that the insights gleaned are both robust and reliable.
Assess Outputs Critically
Researchers must critically evaluate the outputs generated by synthetic respondents, especially in areas where bias is likely or data diversity may be insufficient. This involves a thorough examination of the assumptions underlying the synthetic models, as well as an assessment of how well the data represents the target population. By scrutinizing the results for potential biases and gaps, researchers can identify and address any distortions or oversights, ensuring that the conclusions drawn are valid and reflective of reality.
Ensure Transparency and Traceability
Maintaining transparency and traceability in the responses generated by synthetic respondents is essential for accountability. Researchers should ensure that each response can be traced back to its underlying data sources, allowing for a clear understanding of how conclusions were reached. This level of transparency not only bolsters the credibility of the research but also enables other researchers to replicate or challenge the findings, fostering a culture of openness and rigorous inquiry.
Respect the Limits of Synthetic Users
It’s important to acknowledge that “synths” are tools that enhance, not replace, traditional market research. They offer significant advantages in terms of efficiency and can handle large volumes of data with ease. However, they lack the depth of understanding and the ability to capture the full spectrum of human experiences and emotions that traditional methods, such as interviews and focus groups, can provide. Researchers should leverage synthetic respondents to complement and enrich their research efforts rather than viewing them as a standalone solution.
Consider the analogy of in silico computational tools used in drug discovery. These tools, which simulate new molecules, have become an invaluable asset alongside traditional experimental methods. They streamline the drug discovery process by refining and narrowing down the hypotheses that need to be tested in actual trials. Similarly, synthetic respondents act as a preparatory tool in market research. They help ensure that researchers are asking the right questions and focusing their real-world studies efficiently, thereby complementing the traditional research process. Just as in silico models do not replace the need for real-world testing in drug development, synthetic respondents should be seen as a means to enhance the depth and relevance of market research findings.
Creating Effective and Accurate Synthetic Respondents
Creating effective and accurate synthetic respondents involves a combination of advanced technology, comprehensive data sets, and a thoughtful approach to minimize biases. Here are key steps and considerations to ensure the synthetic respondents you create are both an effective tool and reflective of the target population:
1. Gather Comprehensive Data
Collecting a wide range of data through surveys, interviews, and existing research is crucial. This diverse dataset forms the foundation for generating synthetic profiles that are realistic and relevant to your study. The comprehensiveness of this data directly impacts the questions you can feasibly pose to your synthetic users, making representativeness vital.
2. Create Detailed Personas
Analyze the collected data to develop detailed personas representing your target population’s various segments. These personas should include specific attributes, behaviors, and preferences, providing a nuanced view of the demographic you’re studying.
3. Select Appropriate AI Tools
Choose AI and machine learning technologies capable of processing the collected data and simulating human responses. Feed the collected real-world data into your AI model to train it. The quality and diversity of the training data are crucial for the accuracy of the synthetic respondents.
4. Test Against Real Data
Validate the responses of your synthetic respondents by comparing them with real data or feedback from actual participants. This helps identify any inaccuracies or biases. Continuously refine your synthetic respondents based on feedback and new data. This iterative process enhances their realism and accuracy over time.
5. Ensure Ethical Considerations and Transparency
Be transparent about the use of synthetic respondents and consider the ethical implications. Maintain a clear traceability of how conclusions are drawn, allowing for validation of the synthetic respondent’s utility and the explanation of its reasoning to stakeholders.
6. Deploy in Research Studies
Integrate synthetic respondents into your market research projects, using them to complement traditional research methods. Regularly assess how well synthetic respondents are meeting your research objectives and make adjustments as necessary.
Getting Started with Synthetic Respondents
Creating synthetic respondents or personas for market research can be approached in several ways, each offering distinct benefits, challenges, and applicability. Below is a comparison of a few potential strategies:
Utilizing Tools like ChatGPT or Gemini
One method involves utilizing tools like ChatGPT, Gemini, or Character.ai where researchers can engage with pre-trained models directly, using prompts to simulate specific personas. This approach is highly accessible, requiring no technical expertise in AI or coding, making it a cost-effective and flexible option. However, it comes with limitations in customization and is subject to the constraints of the model itself.
Developing a Custom GPT
Another strategy is to develop a custom GPT on ChatGPT, which involves training a model on a specific instruction set or dataset tailored to the researcher’s needs. This method allows for high levels of customization and specificity, leading to more accurate synthetic personas and more control over the training data, including the inclusion of proprietary or niche content. Despite these benefits, it requires a significant time commitment to develop and test a custom instruction set and ensure that you are not injecting inappropriate biases into your GPT.
Leveraging a Third-Party Platform
Alternatively, partnering with a third-party platform that specializes in providing synthetic respondents can lessen the time commitment and technical expertise required from the researcher’s end. However, this option can be more expensive, especially for large-scale or highly customized projects, and may involve sharing potentially sensitive data with an external party. Steps must then be taken to ensure the platform can either remove the data afterward or that the researcher retains ownership of the process’s outputs and that all data is kept secure and isolated.
Building Your Own Solution
Finally, building your own solution by creating bespoke synthetic respondents from scratch, leveraging custom applications, and specific LLMs, offers complete control over the model’s design, training data, and output. This approach allows for precise customization but requires deep expertise in AI, machine learning, and coding, making it a challenging endeavor for those without a technical background. It also demands substantial time and resources for development, testing, and maintenance. Finally, this approach, most of all, is prone to biases that can be hard to remove or worse yet even notice as you are developing your “synths.”
Each method presents a distinct pathway with its own considerations, and the choice among them will depend on the project’s requirements, scale, desired level of customization, available resources, and technical expertise.
Practical Applications and Use Case Examples
The practical applications of synthetic respondents span from creating detailed simulations of individual interviews to facilitating large-scale quantitative studies. Here’s an expanded view on their practical use cases:
Simulating Individual Interviews
By emulating the personality and response style of specific individuals, AI models can simulate one-on-one interviews. This approach allows researchers to interact with an AI-generated persona as if they were conversing with a real person.
Such simulations are particularly useful for understanding the perspectives of key influencers or target customer groups. Companies can delve into how these personas might perceive or react to different topics or questions, offering deep insights into individual thought processes and decision-making criteria. This technique is invaluable for tailoring marketing strategies or product designs to meet the nuanced preferences of influential figures or crucial demographic segments.
Conducting Large-Scale Quantitative Research
Synthetic personas can be created using real survey data from a wide range of individuals, allowing for the simulation of diverse responses across large sample sizes, such as 1,000 or 10,000 respondents. This method provides the ability to analyze broad market trends, preferences, and behavioral patterns.
Leveraging synthetic respondents in this way effectively sidesteps the logistical challenges typical of traditional survey methods, such as the need for participant recruitment and the complexities of data collection.
A recent study by Cornell University exemplifies this approach. The researchers developed “silicon samples” by conditioning a model on thousands of socio-demographic narratives derived from real human participants across several extensive surveys in the United States. By comparing these silicon samples with actual human data, the study found that the insights generated by their synthetic sample extend well beyond mere surface-level similarities. The findings were nuanced, multi-dimensional, and captured the intricate relationship between ideas, attitudes, and the socio-cultural framework that shapes human perspectives.
Augmentation
Synthetic respondents serve as an excellent augmentation to conventional research methodologies, offering initial insights or helping to define the scope of research projects. As noted by Gartner, the trend among companies is to emphasize the role of synthetic respondents as a complementary tool rather than a standalone solution.
Synthetic Respondents and the Future of Market Research
Synthetic respondents are rapidly transforming market research efforts. Researchers who skillfully embrace “synths” while ensuring their limitations are well understood are well positioned to provide critical insights for their clients today and long into the future.
In sum, “synths,” when leveraged safely and effectively, merely jumpstart our understanding and in no way should be seen as a threat, but merely as a meaningful complement to traditional research methods.
“Some people worry that artificial intelligence will make us feel inferior, but then, anybody in his right mind should have an inferiority complex every time he looks at a flower.”
—Alan Kay – An American Computer Scientist best known for his pioneering work on Graphical User Interfaces (GUI) which led to the first modern “windowed” computer interface.
About the Authors
The content and insights found on this page were developed as part of a joint effort between Cascade Insights and Coloop. Specifically, contributions were made from:
To learn more about Coloop and how you can use AI tools to enhance qualitative research efforts, reach out to Jack at [email protected].
B2B tech companies seeking deeper insights into their market, customers, or competitors can contact Cascade Insights to learn more.
Few of our Clients
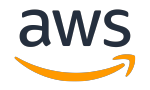
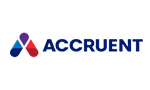
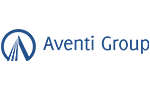
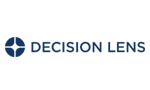
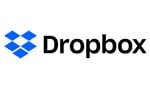
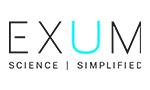
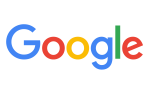
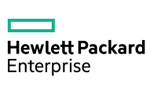
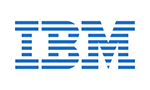
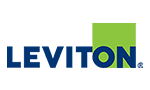
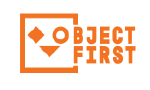
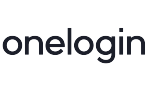
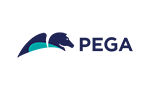
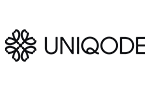
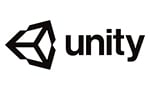
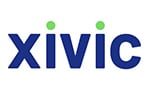
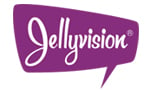
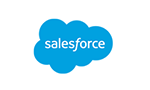